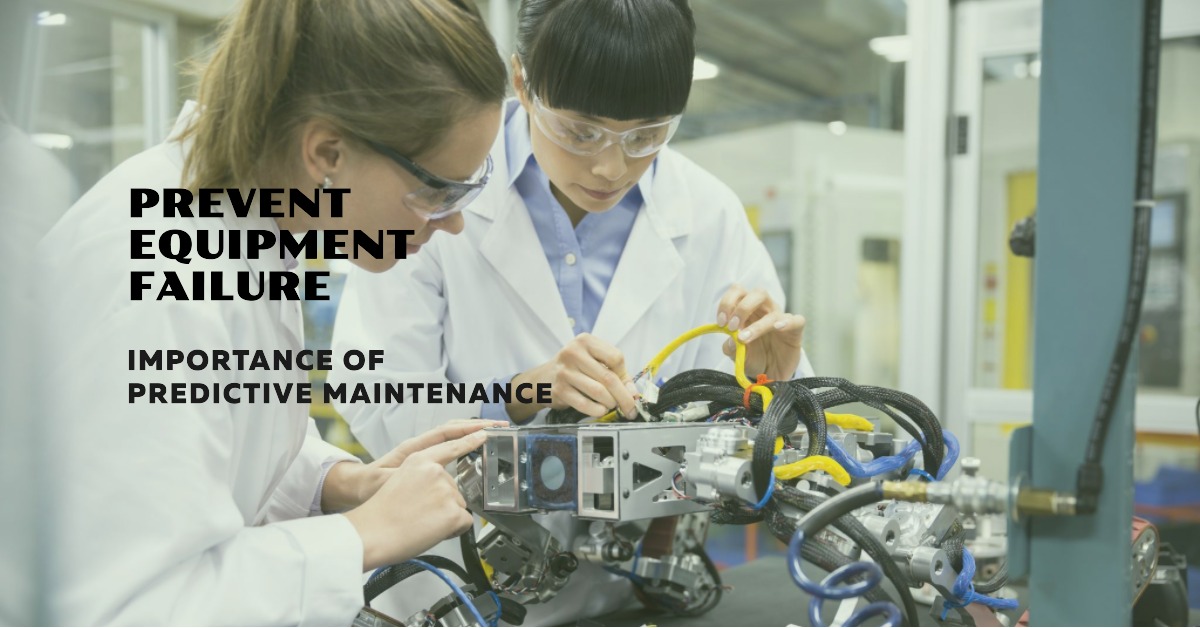
In an industrial setting, efficiency and productivity reign supreme. As such, any form of equipment downtime is not just an inconvenience—it's a significant financial loss. Enter: Predictive Maintenance. This smart, data-driven approach to maintenance is transforming industries worldwide, revolutionizing how we manage and maintain machinery for optimal performance. It's the service of the future made available today.
Predictive Maintenance (PM) essentially leverages machine learning and IoT technology to monitor equipment and predict potential breakdowns before they occur. This significantly reduces the likelihood of unexpected failures and the consequential large losses due to downtime in manufacturing companies.
The initial process involves monitoring complete plants and production lines remotely to capture important data, which are then processed and analyzed using machine learning algorithms. The model built from these algorithms can then predict potential machine failures, allowing for planned maintenance activities and averting abrupt disruptions.
The Power of Data in Predictive Maintenance
"Big Data" and "Predictive Maintenance" go hand in hand. The concept of predictive maintenance thrives on the capability to process vast amounts of data in real-time, a feature facilitated by big data. It's no wonder that in 2019, big data was one of the key trends in after-sales service.
Data derived from sensors placed on equipment provide insights into the equipment's working condition. These real-time data streams, combined with historical data, provide a comprehensive overview of machine health. Hence, any unusual patterns or anomalies that might signify a future breakdown can be detected early on.
The Role of Artificial Intelligence
Artificial Intelligence (AI) is the backbone of predictive maintenance.
AI's ability to process and analyze vast amounts of data in real-time makes it integral to the functioning of predictive maintenance systems. Artificial Intelligence in manufacturing has come a long way, especially with advancements in machine learning algorithms and computational power.
By recognizing complex patterns in data that might be overlooked by manual analysis, AI can accurately forecast when a machine is likely to fail. This is, however, only the tip of the iceberg when it comes to the potential applications of AI in customer service.
The Impact of Predictive Maintenance
Predictive maintenance is rapidly becoming an indispensable component of modern industries. Its advantages go beyond mere maintenance. For instance, PM plays a significant role in enhancing customer service by ensuring minimal disruptions to operations.
Industries that have implemented predictive maintenance have observed a considerable decrease in maintenance costs, along with an increase in equipment life and operational efficiency. This is not to mention the significant strides made in improving customer service processes.
In conclusion, predictive maintenance, powered by AI and big data, holds the promise of transforming industrial maintenance from a reactive task to a proactive strategy. With its ability to predict equipment failures, it is paving the way for more efficient, cost-effective, and reliable operations in the industry.
Harnessing the Technology: The How of Predictive Maintenance
While Predictive Maintenance (PM) is a buzzword today, how does it actually function? What are the integral technologies that make it possible? Let's dive deeper.
The IoT & Sensors
One of the significant contributors to the rise of predictive maintenance is the Internet of Things (IoT). IoT, in combination with sensor technology, enables real-time monitoring of equipment and machinery across multiple parameters. It's these smart services that lay the groundwork for PM.
Sensors placed on equipment relay real-time data about factors such as temperature, vibration, and humidity, which are indicative of the machine's health. The IoT connects these sensors to a central database, enabling seamless and continuous data transmission.
Cloud Computing
With large volumes of data being generated, it is imperative to have a robust and secure system for storage and processing. This is where cloud computing comes into play. Cloud-based platforms not only offer scalable storage solutions but also provide the computational power required for complex data analysis and machine learning algorithms.
Machine Learning
At the heart of predictive maintenance is Machine Learning (ML). ML models can identify patterns in the data collected and learn from these patterns to make predictions. Machine Learning, particularly when used in after-sales service, can not only predict possible equipment failures but also optimize servicing schedules based on usage patterns and anticipated life cycles.
Digital Twins
A more recent development in the field is the use of digital twins – virtual replicas of physical assets. The digital twin in the manufacturing industry can simulate a machine's performance under different scenarios, thereby offering a more comprehensive view of possible outcomes.
Augmented Reality
Augmented Reality is another tool making waves in the field of maintenance. AR can superimpose computer-generated images onto a user's view of the real world, providing composite views that augment the understanding of how systems work or how they might fail.
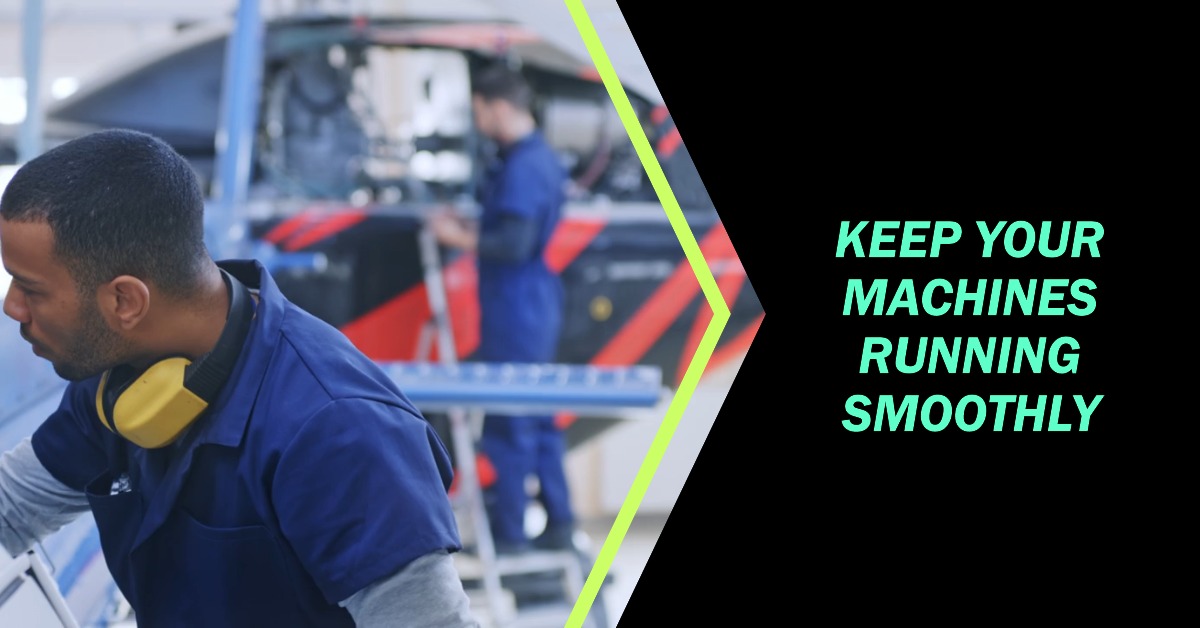
Implementing Predictive Maintenance
Implementation is the key to unlocking the potential of Predictive Maintenance.
Moving from reactive or preventative maintenance to predictive maintenance is no small feat. It requires strategic planning, a clear understanding of your equipment and its failure modes, and technological integration.
One of the first steps to implementing predictive maintenance is creating a ticketing system to log all machinery incidents. This will become a valuable source of data for your predictive model.
Predictive Maintenance Challenges
Although promising, predictive maintenance is not without its challenges. One of the major challenges is managing and analyzing the massive amounts of data generated. Digitization in manufacturing is a vast domain and data management is at the heart of it.
Another significant hurdle is the integration of predictive maintenance technology with existing systems. It's important to have a detailed implementation strategy to ensure a smooth transition.
Data Management
Effective data management is crucial for predictive maintenance. Utilizing cloud-based platforms, AI and machine learning, data can be effectively managed and utilized. To this end, the integration of AI into customer service platforms can play a pivotal role in managing, sorting, and making sense of the massive amounts of data generated. Our post on AI in customer service sheds light on the potential benefits and practical applications of AI in this realm.
Integration with Existing Systems
Incorporating predictive maintenance into existing systems is another hurdle. A phased implementation approach can make the transition smoother and more manageable. Start with areas where predictive maintenance will deliver the most impact, such as high-value or high-risk equipment. Our post on implementing a customer portal provides some strategies that can be beneficial in the context of predictive maintenance implementation.
Future Developments
As technology advances, we can anticipate the rise of more sophisticated predictive maintenance techniques. One such development could be the integration of artificial intelligence in manufacturing. With AI, predictive models can become even more accurate, and the scope of predictive maintenance could expand significantly.
Moreover, with the advancements in AR, remote assistance can provide real-time support and maintenance instruction overlaying on the physical equipment. Such smart and futuristic technologies can revolutionize predictive maintenance.
Conclusion
Predictive Maintenance stands at the intersection of IoT, cloud computing, machine learning, and many other cutting-edge technologies. It represents a significant leap in asset management, transforming how we approach maintenance, and promises immense potential for companies willing to invest in it.
The road to predictive maintenance may have its challenges, but with careful planning, strategic implementation, and the use of advanced technologies, these hurdles can be overcome, leading to streamlined operations, improved efficiencies, and considerable cost savings.
With Predictive Maintenance, the future of maintenance is not just about fixing what's broken, but predicting what could break and preventing it.
FAQ
-
What is predictive maintenance?
Predictive maintenance involves the use of data-driven, proactive maintenance strategies that are designed to predict when maintenance should be performed. This approach offers cost savings over routine or time-based preventive maintenance because tasks are performed only when needed.
-
How does predictive maintenance work?
Predictive maintenance uses condition-monitoring equipment to evaluate the performance of machinery and equipment. It utilizes data from machine sensors to determine when a machine will likely fail. Machine learning and AI algorithms are often used to predict equipment failure and to determine the optimal time for maintenance.
-
What are the benefits of predictive maintenance?
Predictive maintenance can result in significant cost savings because maintenance is performed only when necessary. It can also reduce equipment downtime, improve resource allocation, and increase operational efficiency.
-
What challenges does predictive maintenance face?
Challenges include effective data management, integrating with existing systems, and overcoming skepticism from those used to traditional maintenance methods. Additionally, predictive maintenance requires a shift in organizational culture to a more proactive mindset.
-
What is the future of predictive maintenance?
The future of predictive maintenance lies in the increased integration of AI, IoT, and other technologies. This will allow for more accurate predictions, more efficient processes, and broader applications.
-
How can AI support predictive maintenance?
AI can support predictive maintenance by analyzing large quantities of data to identify patterns and predict equipment failure. This can lead to more accurate maintenance predictions and a reduction in unnecessary maintenance.
-
What role does AR play in predictive maintenance?
AR can provide real-time, hands-on training and assistance to maintenance personnel, potentially even allowing experts to guide procedures from a remote location. This can reduce error rates and improve the efficiency of maintenance tasks.
-
What are some real-life applications of predictive maintenance?
Real-life applications can be found in numerous industries, including manufacturing, energy, transportation, and healthcare. For example, a manufacturer might use predictive maintenance to monitor high-value or high-risk equipment, preventing unexpected downtime.